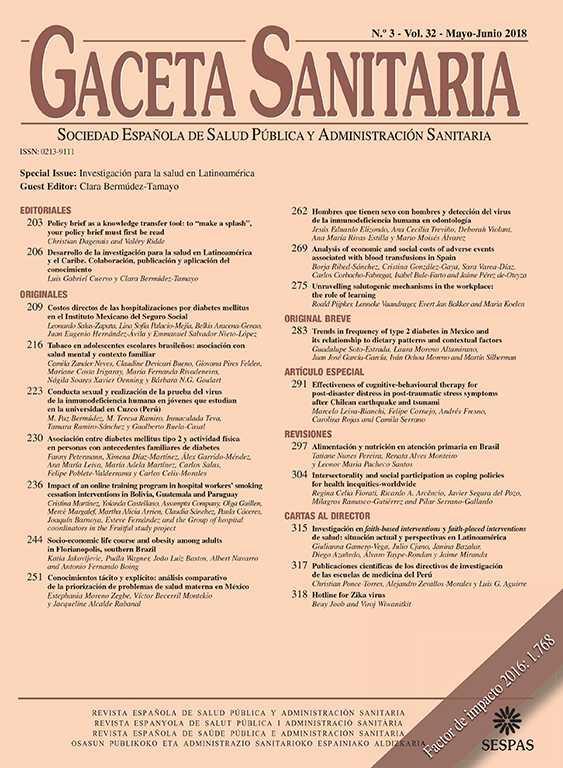
The 3rd International Nursing and Health Sciences Students and Health Care Professionals Conference (INHSP)
More infoThe COVID-19 pandemic put enormous socio-economic pressures on most countries all over the world. In order to contain the spread of the coronavirus, governments implemented both pharmaceutical and non-pharmaceutical interventions. This simple modeling work aims to quantify the effect of three levels of social distancing and large-scale testing on daily COVID-19 cases in Malaysia, Republic of Korea, and Japan.
MethodThe model uses a Stepwise Multiple Regression (SWMR) method for selecting lagged mobility index and testing correlated with daily cases based on a 0.05 level of significance.
ResultThe models's predictability ranges are from 75% to 92%. It is also found that the mobility index plays a more important role, in comparison to testing rates, in determining daily confirmed cases.
ConclusionBehavioral changes that support physical distancing measures should be practiced to slow down the COVID-19 spreads.
Since its first outbreak on late December 2019 from Wuhan China, Coronavirus Disease 2019 (COVID-19) spreads rapidly and brings enormous socio-economic impacts to affected countries in the globe. As of 25 June 2020, more than 9.4 million cases and 482 thousand deaths are reported worldwide.1 These numbers still seem to escalate over time and affect more areas. In order to suppress further spread of this virus, WHO recommends each country to follow six steps: increase public health workforce capability, construct a tracing system for detection, build-up testing capacity and availability, identify and adapt key facilities for medical treatment, conceive a clear plan to quarantine contacts and refocus national policy on controlling COVID-19 spread.2
In responding to this pandemic Governments around the world have implemented a range of strategies such as: containment, mitigation and suppression. Widespread social distancing measures, quarantine, and lockdowns are favored by some countries,3 while others prefer to initiate large-scale testing and tracing strategies.4 The outcomes of these efforts in virus containment are measured by the so-called effective reproduction number, Reff. There are three regimes or levels of social distancing performed in many countries and their effectiveness has not been compared yet.5,6 It is important to note that these policies were successful in controlling the prior epidemics.7–9
Social distancing will limit the mobility of people. The main target is those with symptomatic and asymptomatic virus carrier to prevent further transmission in a community. COVID-19 testing is conducted to identify whether or not a person has the virus. The test's result will trigger other procedures, i.e. contact-tracing and isolation. So, both social distancing and testing are expected to control virus spread. However, their roles are still not clear. This work presents a simple multiple regression model quantifying significant factors responsible for COVID-19 cases in three countries with different level of social distancing. The work is expected to contribute in shaping policies for pandemic containment.
MethodDataDaily confirmed cases of COVID-19, testing rates and social distancing measures from three countries are retrieved from the Institute for Health Metrics and Evaluation website. The regimes are classified as: all closed, partly closed, and educational closed and they are further quantified as mobility index. These different regimes are represented by Malaysia, Republic of Korea, and Japan, respectively. All data set is downloaded and presented in Supplementary data: COVID-19 data. The model inputs or predictors consist of lagged in time for both testing data and mobility index. The lagged days starts from the day of testing to the previous 14 days before the testing event. These lags are written as subscript ‘0’, and ‘−14’, respectively (see Table 1). This lagged in times is selected due to the maximum incubation period of Coronavirus reported by WHO,10 which then used by the Governments for determining the length of quarantine.11
SWMR model using lagged Mobility Index (MI) lagged Testing (T) and their associated skill.
Country/period | Predictors | Models | Prediction skills | |
---|---|---|---|---|
Predictability R2 (%) | RMSE (cases) | |||
Malaysia: 01/03/2020 – 12/05/2020 (73 days) | MI-4 (β=3.937), T-14 (β=0.023), T-8 (β=0.006), T-14*MI-7 (β=0.001) | Daily cases=−4.491*MI-4+0.031*T-14−0.007*T-8+0.001*T-14*MI-7−29.375 | 75 | 32 |
Rep. of Korea: 20/02/2020–13/05/2020 (84 days) | MI-1 (β=13.832), T-5 (β=0.024), T-5*MI-1 (β=0.004) | Daily cases=89.669*MI-1−0.183*T-5−0.011*T-5*MI-1+1527.6 | 84 | 75 |
Japan: 17/02/2020 –10/05/2020 (84 days) | MI-1 (β=151.287), MI 0 (β=92.173), MI-4 (β=72.858), MI-9 (β=29.384), MI0*MI-9 (β=1.247) T-5 (β=0.153), T-1 (β=0.074), T-5*MI-4 (β=0.008) | Daily cases=−167.82*MI-1+105.8*MI0+71.14*MI-4−31.28*MI-9−1.14*MI0*MI-9−0.17*T-5+0.134*T-1−0.006*T-5*MI-4−120.61 | 92 | 58 |
In this study, we apply Stepwise Multiple Regression (SWMR), the combination of forward selection and backward elimination method to select significant lagged test and mobility index that correlate with confirmed COVID-19 cases. The SWMR selects the significant inputs or predictors based on p-value of 0.05. The resulting selected inputs are presented in Table 1.
Stepwise regression uses the same analytical optimization procedure as multiple regression but differs in that a subset of predictor variables is selected sequentially from a group of predictors using statistical testing or hypotheses. This procedure rely on the Gauss–Jordan algorithm, whereas the optimum or ideal sub-model chosen from a candidate model containing only variables that are statistically significant at a specific level.12 This method has been used in many applications, such as marine and fisheries,13 mining and geology,14 engineering15 atmospheric studies16 even social and psychological research.17
Model skill assessmentThe skills of models in predicting daily confirmed cases of COVID-19 for each region are measured using predictability, i.e. the coefficient of determination R218 and root-mean squared error (RMSE). The modeling scheme and their skill outputs in each social distancing regime are presented in Fig. 1 and Table 1.
SWMR (Stepwise Multiple Regression) model for selecting significant predictors associated with COVID-19 confirmed cases. The input consists of daily testing, and daily-lagged data for both the number of test performed and mobility index. The prediction skill of the models is summarized in Table 1.
The prediction skill of SWMR model is summarized in Table 1. Table 1 shows significant factors, determined by their standardized coefficient β’s, which are responsible for daily confirmed cases of COVID-19 in three social distancing regimes.
Each country has different significant factors in determining the daily COVID-19 cases. Malaysia, for example, applies a full closed mode. The significant factors are MI-4, T-14, T-8, T-14*MI-7. Here, the mobility index has a stronger effect than testing efforts in determining COVID-19 cases in view of the standardized coefficient β’s. The Malaysian cases show that there is a negative correlation between COVID-19 cases and the mobility index. If more people stay at home means less social interaction between people. This will result in reducing the confirmed COVID-19 cases.
Republic of Korea or South Korea with partly closed mode has three significant factors MI-1, T-5, T-5*MI-1. South Korea has mobility index as a stronger effect but indicates a positive correlation with COVID-19 cases. South Korea applied mitigation strategies such as large-scale testing and tracing in the early stage rather than suppression. When this country has the second largest confirmed cases in the world, on February 23, the Korean government has raised the COVID-19 alert level to the highest (Level 4) along with the enactment of social distancing measures.19 However, despite the rapid implementation of a massive detection strategy, South Korea is successful in responding the epidemics.4,20
Japan has eight significant predictors dominated by mobility indices, MI-1, MI 0, MI-4, MI-9, MI 0*MI-9, T-5, T-1, T-5*MI-4. Again, the mobility index is more important than that of the number of test conducted in determining COVID-19. Japan conducts their own model in response to COVID-19, using the cluster-based approach and behavioral changes that mostly related to Japanese tradition. Japanese has the habit of wearing masks and practicing the non-physical contact etiquette 21 and social distancing measures.22
Of three social distancing regimes, it's demonstrated that the mobility index plays an important role compared with testing, despite each model consists of coupled factors combining both. This result seems to assert the nature of SARS-CoV-2 as high transmissibility virus which has a range of basic reproduction number from 2.8 to 5.5 in the absence of full quarantine and social distancing measures.23 Experience from past epidemics, in the absence of effective intervention and supportive healthcare system, a variety of non-pharmaceutical interventions are proven to successful in reducing case numbers.23 It means that isolating, quarantine, contact tracing, social distancing, travel restriction, non-essential facility closure, and avoiding all activities in a large group have shown to be effective ways in responding to the outbreak when implemented early in the epidemic.23–25
Fig. 2 shows the comparison between observed and the predicted daily confirmed COVID-19 cases. Note the different range of time depending on recorded data for each country. Note also that, each country has its own epidemic curve. For instance, South Korea has its cases peaks in late February while Japan just entered its peak at the beginning of April. Even though the model's predictability is above 75%, the model fails to predict the flat peak in Malaysia, the highest peak-level in Korea and decreased cases in Japan.
ConclusionsAll models predictability for the three countries with different social distancing regimes are above 75%. The mobility index plays more important role than the number of testing in determining COVID-19 cases.
Supplementary data: covid-19 dataThe supplementary data can be found on this github repository: Https://github.com/andika9807/supplementary_data_mobility.
Conflicts of interestThe authors declare no conflict of interest.
We thank the institute for health metrics and evaluation for providing the dataset of daily confirmed cases of covid-19, daily covid-19 tests, and the mobility indices of Malaysia, South Korea, and Japan available to the public.
Peer-review under responsibility of the scientific committee of the 3rd International Nursing, Health Science Students & Health Care Professionals Conference. Full-text and the content of it is under responsibility of authors of the article.