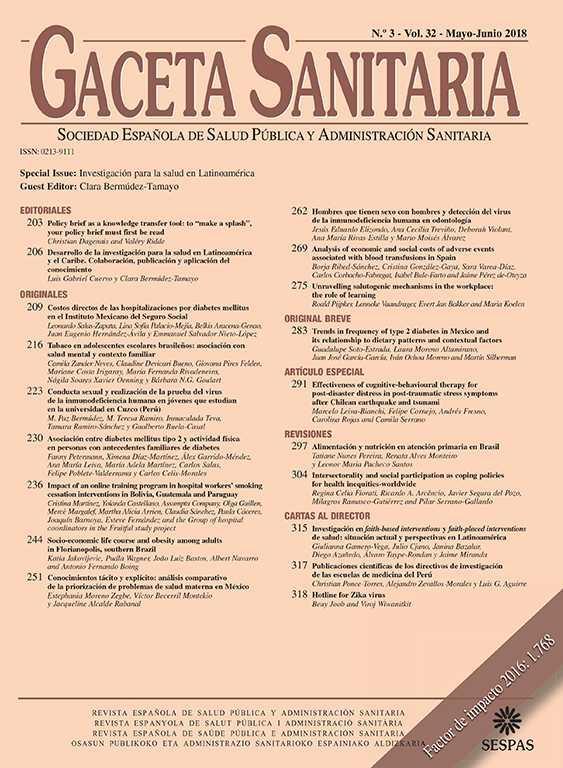
The 3rd International Nursing and Health Sciences Students and Health Care Professionals Conference (INHSP)
Más datosTo identify the method used in detecting fraud cases.
MethodsArticles searching by using topic-appropriate keywords and incorporated into search engines (data-based) journals Pubmed/Medline, Cochrane, Wiley, ScienceDirect, and secondary data-based Google scholar. Then data extraction is done based on inclusion criteria. The selected articles have the aim of investigating/detecting cases of fraud that have occurred in the health sector or other related sectors that support the study.
ResultsThe findings of the nine reviewed articles have suggested that most of the fraud perpetrators are performed by medical personnel (doctors) and providers. Many types of fraud occur such as insurance claims or medical actions that are completely unadministered nor following the procedure and duplicating claims. The methods that appropriate to be used in detecting fraud are secondary data tracking, information, and technology specialist provision.
ConclusionSecondary data tracking is the most widely used method in fraud detection. Fraud perpetrators are ones who dominated by medical circles with fictitious claim cases. Perpetrators tend not to act themselves but in organizations with network.
Fraud on health care has been classified as an international challenge as well as illegal actions, where perpetrators create a zero-sum game for maintenance costs that contribute to the cumulative effect on the quality of service.1,2 Estimated Fraud costs up to 10% of total health care expenditure worldwide.3 In the United States from 3.6 trillion dollars incurred for health care costs, billions of dollars are claims for fraud.4 Viewing RGA data (2017) the state of Asia Pacific that responds to fraud event data including Australia, Singapore, Malaysia, Thailand, the Philippines, Vietnam, Japan, Hong Kong, Korea, Taiwan, and Indonesia.5 The Data indicates there are opportunities of various stakeholders in the act of fraud, not limited to state conditions both developed and developed.
Fraud is building a serious threat that disrupts the global economy with the extravagance of unnecessary or counterfeit maintenance costs and adverse health consequences, so it takes solving appropriate problems in their finishing.6,7 Fraud in the field of health occurs and continues to increase in South Africa.8 The European continent has at least €56 billion losses annually over fraud practices. However, the data is only reported from some countries in Europe, some of which deny the problems related to fraud with privacy assumptions.9 Fraud issues also occur in Korea and continue to increase, reportedly at least 798.2 billion won claimed in 2018 or up 9.3% from the previous year.10
These conditions and situations have an impact on policymakers, anti-fraud, and practitioner difficulties to find reliable evidence and keep abreast of the literature review that has been published in a variety of different formats and references. Therefore, comprehensive synthesis and evaluation are critical to providing useful information and reliable evidence to decision-makers who can be gained through systematic review reviews as an efficient solution to address these issues.
Literature reviewThe fraud case impacts the increase in its main cost to everyone involved in the healthcare system and damages the long-term solvency of programs such as insurance services/health care plans in the underprivileged community (Medicare/Medicaid) on millions of people in the Americas (Baranek, Action, & Services, 2018).11 Anti-fraud agencies have tried to detect those frauds and it is strongly suspected that they do not act on their own but have a network like an organization.1,2,4,5
The review of the literature aims to review the results of published studies/reviews and gather the best evidence, then summarizes the evidence and current knowledge regarding the effects of intervention or policy from various sources.12 Our review aims to collect, evaluate, and synthesize evidence from a variety of published literature reviews on fraud detection that occur in the areas of service and health insurance, to provide reliable evidence and enable researchers, policymakers, and practitioners to make informed decisions. The quality flaws in the literature review are also identified, which can be used in formulating recommendations for better quality future reviews.
MethodsLiterature search strategyPreceded by entering the keyword “Fraud” AND “Fraud detection OR Method Fraud” AND “Health Care” on some search engines (data-based) such as Pubmed/Medline, Cochrane, Wiley, Sciencedirect and secondary data-based Google scholar.
Inclusion and exclusion criteriaInclusion criteria: A study that has been studied and written in English and accessible, the article is a reference listed as the international reputable journal web of Science/Thomson Reuters Journal or Scopus, as well as the journal, has a purpose to identify the methods used in detecting fraud that occurs in the field of service/health care. The criterion for extraction exclusion is a journal related to the theme and sub-theme in which include understanding the concept of fraud but published over the last 10 years since the study of a literature review is conducted, only abstract (non-full-text), literature study results or systematic review/meta-analysis.
Data extraction. In this literature review, the data is conducted using a flowchart prism (Fig. 1), and the article that is the inclusion of the feasibility check is done by using the STROBE instrument.
ResultsFindings in the literature review of this library found nine articles that were later reviewed through the synthesis of grids, of which from the 10 articles the findings are largely focused on the detection method of fraud.
Identification of types and perpetrators of fraudFive out of nine articles reviewed discussing the types and perpetrators of fraud; Most of the perpetrators of fraud in the field of health care are medical personnel physicians.13–15 Other fraud perpetrators are also conducted by the provider/healthcare provider, medical member schemes including family practitioners and psychiatrists, employees, companies, and related syndicate.13,15 The average fraud perpetrator is about 21–43 years old.16 The type of fraud done, for example; The Act of Abuse of authority/profession performed by a medical physician,14 filing false claims, irregular claim codes, recurring claims, making fictionalized cards, and claiming services not done.13,16 Besides, it is also found the impact that fraud can cause, such as; Detrimental to the healthcare industry,14 and the results of the study found that around 40% could harm patient care and safety.15
Fraud case detection method in health serviceThere were six of the nine articles reviewed, of which the six articles found that there were several methods in detecting potential fraud, for example; Provide professionals/experts/Technology Information experts (IT) who can detect data fraud.17
A claims detection system and the incorporation of a health insurance/Medicare service DataSet.16 Perform comparative data tiers and a detailed algorithm-level benchmarking method at every data class level.18,19 Then, the method with comparison of recorded data/documented in the document book with electronic data, and proven electronic/digital data more significantly detected fraud case that occurred.20 Also, unisex methods/Special grouping strategies, transfer and isolation of data classes are applied in various medical health care specialties.21
DiscussionFraud is increasingly rampant in the care and health services industry.22 In connection with the findings on one of the reviewed articles, the privacy laws can be a barrier to managing the organization's scale fraud practices.16 On the other hand, The National Health Care Anti-Fraud Association (NHCAA) has suggested several key factors to guide health fraud (fraud) laws that include the use of predictive modeling and treatment transparency between private insurance companies and government programs (National Health Care Anti-Fraud Association.23. Reimbursement for Medicare (health insurance Services in underprivileged communities) can also reduce fraud and misuse and more potentially improve the quality of care for patients.22
The perpetrators of fraud in hospital health care are much done by medical personnel, staff/employees, or healthcare providers (providers).13–15 Make fictionalized documentation for example ordering improper procedures and performing the actual procedures they are not doing or duplicating a claim.16,17
Holmberg and Rothstein (2011); Kirlidog and Asuk (2012); Bauder et al. (2016) state, that vulnerable fraud is found in various types of insurance including health insurance systems to obtain personal gains and or groups in the form of claims on insurance for health care.24–26 It is in line with a study that says that many Americans are not guilty of being deceived when visiting various medical facilities or when they get medication. Lack of education in understanding different medical information facilitates medical fraud.27 Usually, those who are exploited do not know the fact that the wrong or improper action is taking place. However, this is not always controllable. Because people who perform these actions are professionals in the healthcare industry. They take advantage of innocent people because of the lack of knowledge about fraud.
As for the fraud detection methods can be done by collecting all data on the claim is either data-based documentation on paper or electronic, then compare the data and divide it into a level of certain classes.18,21 In line with the previous study in decoding fraud that can be done by dividing the data into several levels/stages. The methods used in this study divide into five stages, the first three phases are aimed at detecting fraud among providers, services, and the number of claims. Then the fourth stage integrates the information obtained in the previous three stages into the overall risk size. Furthermore, the last stage is carried out decision making calculating the risk threshold value. The final decision to declare whether the claim proved to be an indication of fraud or not.28
Ultimately the purpose of the fraud detection method is to prevent the loss of both financially and inpatient care and health services. Methods can be adjusted according to the condition or problem (fraud) that is being felt. When looking at the results of a review from the synthesis table that has been done in the past most of the studies are taking the secondary data which is then used to determine/claim the fraud event. Therefore, further researchers may be able to identify the weaknesses of the fraud detection method through data collection, the types and rules that apply to the perpetrators of fraud in various countries then compare them, so that it can be used as consideration for legal sanctions affirmation for fraud regardless of the status of the job/profession (Table 1).
Synthesis grid.
No | Title (researcher, year) | Main issues | Characteristics | Research variables | Findings | Advantages | Disadvantages | ||
---|---|---|---|---|---|---|---|---|---|
Subject | Instrument | Method | |||||||
1 | Improving Fraud and Abuse Detection in General Physician Claims: A Data Mining Study14 | There is no there is an increase in the expenditure of health industry in various countries, which is directly proportional to the total health expenditure resulting from the practice of fraud and abuse up to billions of dollars per year including the state of Iran. Potential conflict of interest reported by authors. | General Physician | This research instrument forms observations of data obtained from the Iranian Social Security Organization (SSO). Thirteen indicators were developed in total. 2 cost issue indicators, 4 frequency indicators, and visit patterns, and 7 indicators related to prescription patterns. | Use a statistical engineering data mining approach to identify each dataset of health insurance organizations with a prescription doctor claim, through five steps:1. clarify the nature of problems and objectives,2. data preparation,3. identification of indicators and selection,4. analysis of clusters to identify the perpetrators (doctors), and5. discriminant analysis to assess the validity of the clustering approach. | a. Fraud detectionb. Abusedetection | Most physicians are detected to act on Abuse and a small portion of more than 2% of doctors take part in fraud practices that harm the healthcare industry. | Researchers can explore the beneficial knowledge of thousands of claims through 5 steps of data mining. I.e.1. Understanding the nature of problems2. Understand data and data preparation3. Preparing indicators (features) and form of election4. Grouping5. Discriminant analysis | Researchers are only reviewing the incidence of fraud and abuse on public services or that are handled by a general practitioner, whereas in situations of claim loss or related fraud determination of interventions in patients in the specialist section is also vulnerable. |
2 | Understanding the types of fraud in claims to South African medical schemes13 | Medical schemes play an important role in the funding of private healthcare services in South Africa. However, the sector is in the identification of conducting fraud activities that have a bad impact on the service. | Administrate or medical scheme | A simple instrument used is a recording tool and an interview guide to interview. Data is collected through individual semi-structured interviews. Researchers record audio interviews and copy them into textbooks. | Cross-sectional. Where individuals can provide the most insight and knowledge regarding the type of fraud claims experienced by the medical scheme is chosen as a research participant/sample. | a. Perpetrators of fraudb. Types of fraud activities | The study found that medical schemes are manipulated in a variety of ways either done internally (within the company) or externally. The perpetrators of fraud are healthcare providers, members of medical schemes, employees, brokers and related syndicates.Fraud that is done mostly in submission of false claims, irregular billing of codes, duplicate claims, card making, and claiming services are not provided. | This study carried out slightly different studies with a qualitative methodology approach, which is also claimed as a method that has not been studied in South African medical schemes.The first stage information excavation begins with 3 key informants as well as the stage of the trial in the interview indepth | Researchers have only conducted an exploratory study of the types of fraud in medical scheme claims, without identifying which schemes/parts of the unit might be higher or more fraud actions that could be information materials to take appropriate preventive measures. |
3 | Physician Medicare fraud: characteristics and consequences15 | High Fraud on Medicare insurance with a taxpayer fee of $60–250 billion per year, with the identification of the perpetrator, is a service provider (physician). So two important issues of the basic implementation of the study are:(1) Who is the Doctor of fraud Medicare?(2) What happens after they are caught? | General Physician | Not described in detail the research instruments used but the observation results using data obtained from the office of the Inspector General (“OIG”)-US Department of Health and Human Services (“HHS”). | This research is a secondary data analysis study obtained directly from the office of the Inspector General (“OIG”)-U.S. Department of Health and Human Services (“HHS”). The names of convicted physicians are gathered from public databases.The names are further verified with other public records. The details regarding the demographics and description of the fraud are obtained by searching for court documents, media reports, the Internet, and records made by the American Medical Association and medical licenses. | Doctor's specific actions for Medicare fraud The duration of imprisonment and/or probation is dropped; Amount of fines and/or restitution Sanctions are dropped. | The doctor convicted of criminal Medicare and/or Medicaid fraud tends to be male (87%).Physician practitioners of families and psychiatrists are represented excessively as perpetrators.40% of fraud endangers patient care and safety.37% of doctors convicted of fraud have no imprisonment.38% of doctors with fraud continue to conduct medical practice.6. The average amount of fraud is $1.4 million per convicted physician. 21% are not suspended from medical. It only takes one day for them to be irrespective of fraud. | This article evaluates fairly complete work units of doctors who perform the most fraud and how to apply the law to medical practitioners for fraud | Not assessing the nature or causes of medical practitioners to fraud. |
4 | Financial fraud in the private health insurance sector in Australia: Perspectives from the industry17 | The private health insurance sector in Australia faces challenges in managing fraud and overservicing; Major financial losses, fraud is hard to detect, and resources are insufficient in its completion. | The largest private health insurance manager in Australia. | A simple instrument used is a recording tool and an interview guide to interview. | Qualitative methodological approach. | a. Financial fraudb. Legal force of private health insurance sector | It takes a lot more resources with better IT capabilities in detecting data fraud.2. Privacy laws can be prohibitive to manage organizational fraud practices. | This study carried out slightly different studies with a qualitative methodology approach, which is also claimed as a method that has not been learned much in detecting fraud Abstracts have been clearly outlined in relation to study outcomes. | Researchers only conduct exploratory review of anyone who commits fraud in a medical scheme claim, whether the applicable law or SOP is supportive or not, and other related processes. Without identifying the cause factor in detail. |
5 | Examining the network components of a Medicare fraud scheme: the Mirzoyan-Terdjanian organization16 | Fraud of the United States Medicare system is detected at over $100million. To make losses in the healthcare sector. | Medicare organizations in the US. | Observation of Mirzoyan-Terdjanian organizational data related fraud financial system U.S. Medicare. | An exploratory study using the archive data collection Mirzoyan-Terdjanian, USA. | Fraud schemesNetwork characteristicCentrality | The Medicare system detects the nature of claims over fraud submitted only after hundreds of thousands of dollars in the claim has been paid.In total, there were 70 people involved in this criminal activity, with an average age ranging from 43.21 years. Where from the seventy defendants six are given federal charges separate from the five states.The female group has a very small role in conducting fraud 4. Perpetrators need various networks to conduct fraud practices (not own action). | Do not examine the nature or cause of perpetrators fraud. | |
6 | Big Data fraud detection using multiple medicare data sources19 | In the United States, with the improvement of technology and medical sciences, the quality of public welfare services continues to be attentive and enhanced by the Medicare as an institution that helps manage high cost constraints related to quality healthcare. Therefore, the improvement of this service still has a spotlight on the act of fraud of some parties who seek personal benefit. | Health care centers incorporated in Medicare and Medicaid USA. | Data observation related to fraud data Set CMS (Centers for Medicare and Medicaid Services). | Quantitative research by processing Big Data analyzed on Apache Spark computer system quickly. | Medicare provider Utilization and payment Data:DoctorsPrescriberC. Durable medical equipment, Prosthetics, Orthotics and Supplies (DMEPOS). | There is no way found to know the actions of the perpetrator will commit the fraud in this research especially in the original Medicare, so they recommend using various combined datasets to detect fraud behavior when a physician has submitted a payment through one or all of the evaluated Medicare sections. | The study provides discussion on the processing of Medicare's big data and experiments and exploration analyses to show students and best datasets to detect fraud claimed by Medicare providers. In addition, the processed data sets are regarded as big Data and as such, for their fraud detection experiments use the Spark above the Hadoop YARN cluster that can effectively handle large dataset sizes. | Researchers only detect fraud from secondary data collected, without conducting a deeper study of the fundamental factors of fraud in a public service. |
7 | Medicare fraud detection using neural networks18 | Medicare becomes the best health care access trend today for the American population, especially for the elderly established by the local federal government, but the emergence of data abuse over the practice of fraud burdening tax payments up to billions of dollars and putting the health and welfare of beneficiaries at risk or indirectly hindering the balance of performance. So further detection of problems required. | Health care centers incorporated in U.S. Medicare. | Observation of fraud data Set specific CMS category B of Medicare year 2012–2016. | Research and Development where researchers want to evaluate the cause of service imbalance through 6 levels of data. | Medicare providerUtilization and paymentData of doctors and other equipment suppliers. | The study results showed that using the method Comparing multiple levels of data and learning methods of deep algorithm levels across a variety of class distributions Can detect a more stable fraud than a commonly used basic method. | This research provides evidence that the incidence of fraud can be selected using random over-sampling (ROS), random under-sampling (RUS), and hybrid ROS-RUS. For class imbalance handling on Neural Network based Software defect prediction. | Researchers only detect fraud from secondary data collected, without conducting a deeper study of the fundamental factors of fraud in a service. |
8 | Reducing medical claims cost to Ghana's National Health Insurance scheme: a crosssectional comparative assessment of the paper- and electronic-based claims reviews20 | Year 2003, Ghana introduces the National Health Insurance Scheme (NHIS) to replace the Payment systems directly on primary health services and continue to develop. However, as such advances have increased claims in some districts of the state, by which researchers observe the claim data as of January–December 2014. | NHIS Health Care Center Ghana, Africa | Observation of paper- and electronic-based claims of NHIS in Ghana | Cross-sectional comparative assessment | Document-based claims, and electronic-based claims | 1. There are significant differences in cost adjustment rates between paper and electronic based reviews.2. The data listed in electronic reviews are significantly higher than those recorded from paper-based claims reviews. | The researcher describes the Flow chart of NHIA claims review and reimbursement process as a representation of the reader in understanding the process. | Researchers only detect fraud from secondary data collected, without conducting a deeper study of the fundamental factors of fraud in a service. In addition, there are data on the adjustment of claims for outpatient costs and The cost of inpatient in the database cannot be accessed; Thus, this study was unable to assess the level of adjustment based on Health services for two reviews. Likewise, the number of claims marked by the review of claims and related reasons is also unavailable, So studies are unlikely to analyze the ratio of rejection of the two review systems. |
9 | Approaches for identifying U.S. medicare fraud in provider claims data21 | It is reported that there is a harmony between an increase in the elderly population in the United States, with a chronic illness, implying the need for the continuity of healthcare services in the future that will be dating and the need for programs, such as the U.S. Medicare in particular to assist the medical expenses. However, due to health care fraud conspiracy, these programs are experiencing resource issues as well as the quality and accessibility needed Healthcare services. Therefore, evaluation is required for fraud detection related problems. | Track record specialist at Medicare | Observation of Medicare claim dataset part B from 2012 to 2015. | The best basic model, by comparing logistics regression and Multinomial Naive Bayes, is to test for increased fraud detection by U.S. Medicare part B providers. | Fraud rate | Results showed that by using special grouping strategies, class deletions, and class isolation were applied to various medical service specializations, obtaining diverse but effective results in identifying potential fraud, compared to fraud detection via baseline logistic regression models. | In this journal, researchers use approaches to predict which physician specialties are expected based on The type and number of procedures performed. From that approach, they produced a basic model that can be adopted, namely by comparing the logistical regression and Multinomial Naive Bayes, to test and assess some new approaches to improving the fraud detection of the Medicare section providers. | Researchers only detect fraud from secondary data collected, without conducting a deeper study of the fundamental factors of fraud in a public service. |
The perpetrators who are most often involved with fraud are medical personnel (doctors) who are in a medical scheme and provider of health care providers. The most common fraud case is the insurance claim that is not actually done, duplicating the claim, and as such. Ultimately the purpose of the fraud detection method is to prevent the loss of both financially and inpatient care and health services. Methods can be adjusted according to the condition or problem (fraud) that is being felt. When looking at the results of a review from the synthesis table that has been done in the past most of the studies are taking the secondary data which is then used to determine/claim the fraud event. Therefore, further researchers may be able to identify the weaknesses of the fraud detection method through data collection, the types and rules that apply to the perpetrators of fraud in various countries then compare them, so that it can be used as consideration for legal sanctions affirmation for fraud regardless of the status of the job/profession.
Conflicts of interestThe authors declare no conflict of interest.
Peer-review under responsibility of the scientific committee of the 3rd International Nursing, Health Science Students & Health Care Professionals Conference. Full-text and the content of it is under responsibility of authors of the article.